CSV Quality Assurance in 2023: Unveil the secrets to mastering data accuracy, consistency, and integrity, propelling your organization to unparalleled success!
CSV (Comma-Separated Values) files are widely used for storing and transferring data between programs, systems, and organizations. Ensuring their quality is crucial to avoid errors and inconsistencies leading to incorrect decision-making or system failures.
Understanding CSV File Structure
To ensure a CSV file’s quality, it’s essential to comprehend its structure. A CSV file consists of rows, each representing a record, and columns, which hold individual data elements. The first row typically contains column headers and rows with actual data values.
Column Headers and Data Types
Column headers must be descriptive, unique, and free from special characters. Data types should be consistent across columns. For example, if a column holds dates, all entries should be formatted as dates.
Data Validation and Cleansing
Consistency
Data consistency ensures that values within a column follow a standardized format. Inconsistent data can lead to processing errors, incorrect analysis, or invalid results. Establishing rules for data consistency is crucial for maintaining quality assurance.
Completeness
Verify that your CSV file contains complete data records. Missing data can lead to inaccurate conclusions or cause processing issues. Identifying and addressing incomplete data is essential for quality assurance.
Accuracy
To ensure data accuracy, verify that the CSV file’s values are correct and up-to-date. Cross-checking data against reliable sources or performing calculations to validate results can help identify and correct inaccuracies.
Handling Errors and Anomalies
Error Identification
Use tools or scripts to identify errors and anomalies within CSV files. Look for formatting issues, inconsistent data types, missing values, or duplicate records. Flagging and correcting these errors is essential for quality assurance.
Anomaly Detection
Anomalies are unusual patterns or outliers within the dataset. Identifying and understanding these anomalies helps maintain quality assurance by ensuring your data is representative and error-free.
CSV File Validation Tools
Leverage various tools to validate and clean CSV files. These tools can help identify issues, verify data consistency, and maintain quality assurance.
OpenRefine
OpenRefine is an open-source tool for cleaning and transforming data. It provides a user-friendly interface for identifying and addressing issues within CSV files.
CSVLint
CSVLint is an online tool that checks CSV files for errors and inconsistencies. It validates file structure, identifies missing or mismatched data, and generates a report with suggested fixes.
Excel or Google Sheets
Spreadsheet applications like Microsoft Excel or Google Sheets offer data validation, formatting, and transformation features that can help maintain CSV quality assurance.
Version Control
Establish a version control system for your CSV files. Track changes, maintain a history of revisions, and ensure that only authorized users can modify the data. This helps prevent unintentional alterations or data corruption.
Data Backup and Recovery
Implement a data backup and recovery plan for your CSV files. Regularly create copies of important files and store them in a secure location. In case of data loss or corruption, having a backup ensures you can quickly recover and maintain quality assurance.
Implementing Quality Checks and Automated Testing
Quality Check Procedures
Implement procedures for routine quality checks on CSV files. Schedule regular audits to assess data quality and identify areas for improvement. Verify data consistency, accuracy, and completeness using manual and automated methods.
Automated Testing
Leverage automated testing tools to ensure CSV quality assurance. These tools can help detect errors and anomalies more efficiently and effectively than manual processes. Maintain quality assurance throughout the data lifecycle with automated testing.
Data Documentation and Metadata
Data Dictionary
Develop a data dictionary that describes your CSV files’ structure, content, and format. This documentation should include information about column headers, data types, units of measure, and acceptable values. A data dictionary provides a reference for team members, ensuring consistency and adherence to quality standards.
Metadata Management
Metadata is data about data, providing context and additional information about your CSV files. Implement a metadata management system to store, organize, and maintain metadata associated with your data.
This can help users understand the data’s purpose, lineage, and usage restrictions, thereby promoting quality assurance.
Monitoring and Reporting
Data Quality Metrics
Define and track data quality metrics to monitor CSV quality assurance. These metrics include error rates, data completeness, consistency, and accuracy. Regularly review these metrics to identify trends, areas for improvement, and the effectiveness of your quality assurance efforts.
Reporting and Communication
Establish a process for reporting and communicating data quality issues and improvements. Share findings with relevant stakeholders and involve them in decision-making to promote a data quality awareness and accountability culture.
Continuous Improvement
Review and Update Procedures
Regularly review and update CSV quality assurance procedures to ensure they remain practical and relevant. This includes updating data validation rules, refining data governance policies, and incorporating new tools or techniques.
Learn from Mistakes
Embrace a culture of continuous improvement by learning from mistakes and addressing data quality issues as they arise. Share team members’ experiences and insights to help identify and implement best practices.
Quality Assurance Best Practices
- Create and follow a data governance policy outlining standards, roles, and responsibilities for CSV quality assurance.
- Use automated tools to validate and clean data, minimizing human error.
- Establish a regular review process to verify that data remains accurate and up-to-date.
- Train team members on CSV quality assurance techniques and best practices.
- Identify and resolve data quality issues through open communication and collaboration.
Advanced-Data Profiling Techniques
Statistical Analysis
As a CSV quality assurance expert, leverage advanced statistical analysis techniques to gain deeper insights into your data. You can uncover patterns and relationships within your data using descriptive statistics, correlation analysis, and data clustering.
Machine Learning Algorithms
Utilize machine learning algorithms to enhance CSV quality assurance efforts. These algorithms can help identify complex patterns, anomalies, or relationships within the data that might not be apparent through manual inspection or traditional analysis methods. Machine learning can also improve data validation, error detection, and data cleansing processes.
Custom Validation Rules and Scripts
Custom Rules
Develop custom validation rules tailored to your specific data requirements and industry standards. These rules can address unique data quality concerns and ensure compliance with internal or external guidelines.
Custom Scripts
Create custom scripts to automate CSV quality assurance tasks, such as data validation, error detection, and transformation. Scripts can be tailored to your needs and integrated with other tools or systems.
Data Lineage and Traceability
Data Lineage Tracking
Implement a data lineage tracking system to understand your CSV data’s origin, transformation, and usage throughout its lifecycle. Data lineage tracking helps ensure data quality by identifying potential sources of errors or inconsistencies and providing a basis for audits and data provenance verification.
Data Traceability
Establish processes and tools to maintain data traceability, allowing you to track changes, modifications, and usage of your CSV files over time. Data traceability helps ensure data quality by providing an audit trail and enabling accountability for data stewardship.
Data Quality Assurance in Distributed Environments
Distributed Data Processing
CSV files may be generated, processed, and consumed in a distributed data environment across multiple systems or locations. Implement data quality assurance strategies and tools that can work seamlessly in such environments, ensuring data consistency, accuracy, and completeness across all nodes.
Data Integration and Consolidation
When dealing with CSV files from different sources or systems, ensure data quality during the integration and consolidation. Develop robust data mapping, transformation, and validation rules to maintain data quality while combining disparate datasets.
Industry-Specific Quality Assurance
Regulatory Compliance
Understand and adhere to industry-specific regulations and guidelines that govern the use and management of CSV data. Ensure that your quality assurance practices meet these requirements and maintain compliance with applicable standards.
Industry Benchmarks
Benchmark your CSV quality assurance efforts against industry best practices and norms. Continuously evaluate and refine your strategies to align with industry standards and stay ahead of evolving data quality challenges.
Advanced techniques and practices employed by seasoned CSV quality assurance experts include:
- Advanced data profiling.
- Custom validation rules.
- Data lineage tracking.
- Distributed data processing.
- Industry-specific quality assurance measures.
These advanced approaches help ensure the highest level of data quality, enabling organizations to make informed decisions, minimize risk, and maintain compliance with industry standards.
Conclusion
CSV quality assurance is crucial for maintaining data integrity and avoiding costly errors. Maintaining CSV quality assurance requires understanding file structure, data validation, error handling, leveraging tools, version control, data backup, best practices, implementing quality checks, automated testing, data documentation, monitoring, and continuous improvement.
By following these guidelines and promoting a culture of quality assurance, you can ensure the integrity and reliability of your CSV files, leading to better decision-making and reduced risk of errors.
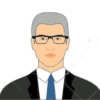
Edward, a distinguished technical writer, is esteemed for his deep knowledge in software testing and quality assurance. Born and raised in the bustling city of Los Angeles, his technological journey is marked with remarkable milestones.
His academic pursuit of computer science at Columbia University in the early 1990s marked the genesis of his career, immersing him in the realms of programming and software development.
Upon graduating, Edward joined Cisco Systems, delving into groundbreaking networking technologies and handling complex software projects. His subsequent role at Bank of America enabled him to enhance crucial financial systems’ stability, security, and efficiency.
Edward later transitioned to Adobe, where he spearheaded robust test strategies, bolstering the user experience of Adobe’s products and reinforcing his position as an invaluable industry asset.
With his unwavering dedication to excellence, Edward is a respected advocate in his field. His journey from Columbia University to industry giants like Cisco Systems and Adobe underscores his commitment to honing his craft. His fervor, expertise, and relentless quest for excellence render him a true pioneer in software testing and quality assurance.